Moksh Jain
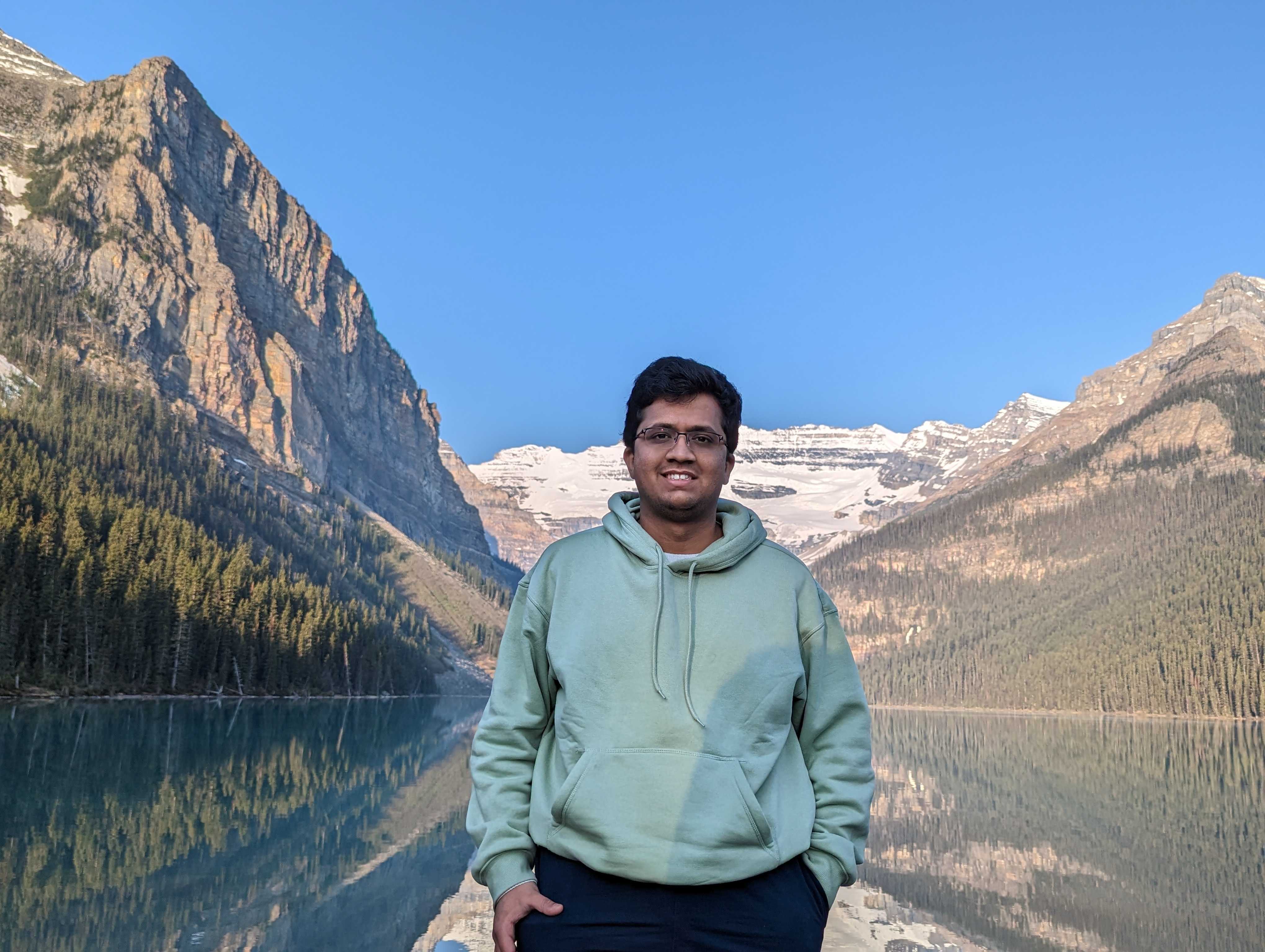
I am a Ph.D. student at Mila and Université de Montréal supervised by Yoshua Bengio. I am interested in developing machine learning algorithms for effective experimental design, incorporating tools from probabilistic inference and modern deep learning. My work focuses on the probabilistic inference framework of GFlowNets. I am interested in applications of these algorithms to accelerate the scientific discovery process. At Mila, I lead various efforts to develop novel machine learning approaches in the context of drug discovery.
I was a research intern with Jason Hartford at Valence Labs (@Recursion) working on experimental design for gene knockout experiments. Before joining Mila as a graduate student, I was a visiting researcher working with Yoshua Bengio on uncertainty estimation and drug discovery. I also spent a year at Microsoft Turing working on compressing and optimizing large langauge models for deployment across Bing and Office.
I completed my bachelor’s degree at the National Institute of Technology Karnataka, Surathkal. To work on my thesis, I spent a semester at Microsoft Research supervised by Prateek Jain and Harsha Simhadri as a part of the EdgeML project, working on efficient machine learning algorithms for resource-constrained environments. I also spent a summer at the Machine Learning group at Leuphana Universitat Lüneburg, supervised by Uwe Dick and Ulf Brefeld.
latest posts
Mar 7, 2023 | GFlowNets and Scientific Discovery |
---|---|
Jul 10, 2019 | Learning Disentangled Representations |